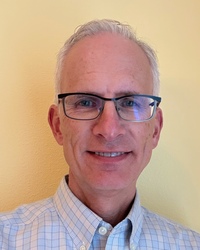
Interests:
Development and application of machine learning and statistical methods for interpreting genomics and proteomics data
Background:
William Stafford Noble (formerly William Noble Grundy) received the Ph.D. in computer science and cognitive science from UC San Diego in 1998. After a one-year postdoc with David Haussler at UC Santa Cruz, he became an Assistant Professor in the Department of Computer Science at Columbia University. In 2002, he joined the faculty of the Department of Genome Sciences at the University of Washington. His research group develops and applies statistical and machine learning techniques for modeling and understanding biological processes at the molecular level. Noble is the recipient of an NSF CAREER award and is a Sloan Research Fellow.
Research:
Research in the Noble lab focuses on the development and application of machine learning and statistical methods for interpreting complex biological data sets. The lab tends to work on research problems that involve fundamental problems in biology while also pushing the state of the art in machine learning. Currently, the lab’s research can be roughly divided into two areas.
- Chromatin and gene regulation. We use motif-based hidden Markov models to characterize collections of transcription factor binding sites in genomic DNA. We also develop models that predict properties of chromatin from genomic DNA.
- Analysis of mass spectrometry data. In collaboration with Michael MacCoss’s lab, we have developed a series of machine learning and statistical methods for the analysis of shotgun proteomics data. In this field, we continue to work on protein identification and quantification, targeted proteomics, and biomarker discovery.
Representative publications:
- Duan Z, Andronescu M, Schutz K, McIlwain S, Kim YG, Lee C, Shendure J, Fields S, Blau CA, Noble WS. “A three-dimensional model of the yeast genome.” Nature 2010;465:363-367.
- Serang O, MacCoss MJ, Noble WS. “Efficient marginalization to compute protein posterior probabilities from shotgun mass spectrometry data.” Journal of Proteome Research 2010;9(10):5346-5357.
- Bailey TL, Boden M, Buske FA, Frith M, Grant CE, Clementi L, Ren J, Li WW, Noble WS. “MEME Suite: tools for motif discovery and searching.” Nucleic Acids Research 2009;37(Web server issue):W202-208.
- Käll L, Canterbury J, Weston J, Noble WS, MacCoss MJ. “Semi-supervised learning for peptide identification from shotgun proteomics datasets.” Nature Methods 2007;4(11):923-925.