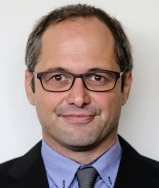
Interests:
Mental health informatics, post-marketing drug surveillance, drug repurposing, analysis of health-related online social media, distributed representations, distributional semantics, and clinical cognition.
Background:
Dr. Cohen trained and practiced as a physician in South Africa, before obtaining his PhD in 2007 in Medical Informatics at Columbia University. His doctoral work focused on an approach to enhancing clinical comprehension in the domain of psychiatry, leveraging distributed representations of psychiatric clinical text. Upon graduation, he joined the faculty at Arizona State University’s nascent Department of Biomedical Informatics, where he contributed to the development of curriculum for informatics students, as well as for medical students at the University of Arizona’s Phoenix campus. In 2009 he joined the faculty at the University of Texas School of Biomedical Informatics, where (amongst other things) he developed a research program concerned with leveraging knowledge extracted from the biomedical literature for information retrieval and pharmacovigilance, and contributed toward large-scale national projects such as the SHARP-C initiative, which supported a range of research projects that aimed at improving the usability and comprehensibility of electronic health record interfaces. Since joining the University of Washington in 2018, he has developed new lines of research concerning detection of linguistic manifestations of neurocognitive status, plain-language summarization of the biomedical literature, and the development of methods to debias deep learning models for natural language processing. He is also an editor of a recent textbook on AI in medicine, published by Springer Nature.
Research:
Dr. Cohen’s research focuses on the development and application of methods of distributional semantics – methods that learn to represent the meaning of terms and concepts from the ways in which they are distributed in large volumes of electronic text. The resulting distributed representations (concept or word embeddings) can be applied to a broad range of biomedical problems, such as: (1) using literature-derived models to find plausible drug/side-effect relationships; (2) finding new therapeutic applications for known (drug repurposing); (3) modeling the exchanges between users of health-related online social media platforms; and (4) identifying phrases within psychiatric narrative that are pertinent to particular diagnostic constructs (such as psychosis). An area of current interest involves applying literature-derived distributed representations in conjunction with observational data as a basis for machine learning. More broadly, he is interested in clinical cognition – the thought processes through which physicians interpret clinical findings – and ways to facilitate these processes using automated methods.
Current projects:
- De-biasing of deep learning models for clinical NLP: On account of their large numbers of trainable parameters, neural language models are vulnerable to biases in which their predictions are influenced by linguistic differences across locations and populations, in addition to language informing the intended primary outcome. The goal of this project is to mitigate these biases, by developing methods to de-confound deep transformer networks – the DeconDTN suite.
- Plain language summarization of the biomedical literature: This project aims to use neural network architectures that are commonly applied to translate between languages to translate the biomedical literature into forms that can be more easily understood by the general public.
- Linguistic indicators of changes in neurocognitive status. Across a range of collaborative projects, our group is developing methods to assess changes in mental state (e.g. indicators of suicide risk and disorganized or distorted thinking), with data sources including transcribed speech, text messages and search logs.
Representative publications:
Bradley, E.* Portanova, J.* Woolley, J. Buck, B. Painer, I. Hankin, M. Xu, W. Cohen, T. Quantifying abnormal emotion processing: a novel computational assessment method and application in schizophrenia. Schizophrenia Research 2024. * indicates joint first authorship.
Guo Y, Qiu W, Leroy G, Wang S, Cohen T. Retrieval augmentation of large language models for lay language generation. Journal of Biomedical Informatics. 2024 Jan 1;149:104580.
Cohen, Trevor A., Vimla L. Patel, and Edward H. Shortliffe, eds. Intelligent Systems in Medicine and Health: The Role of AI. Springer Nature, 2022. https://link.springer.com/book/10.1007/978-3-031-09108-7
Cohen T, Blatter B, Patel V. Simulating expert clinical comprehension: adapting latent semantic analysis to accurately extract clinical concepts from psychiatric narrative. Journal of biomedical informatics. 2008 Dec 1;41(6):1070-87. https://www.sciencedirect.com/science/article/pii/S1532046408000464
Cohen T, Schvaneveldt R, Widdows D. Reflective random indexing and indirect inference: A scalable method for discovery of implicit connections. Journal of biomedical informatics. 2010 Apr 1;43(2):240-56. https://www.sciencedirect.com/science/article/pii/S1532046409001208
Cohen T, Whitfield GK, Schvaneveldt RW, Mukund K, Rindflesch T. EpiphaNet: an interactive tool to support biomedical discoveries. Journal of biomedical discovery and collaboration. 2010;5:21. https://journals.uic.edu/ojs/index.php/jbdc/article/view/3090
Widdows D, Cohen T. Reasoning with vectors: A continuous model for fast robust inference. Logic Journal of the IGPL. 2015 Apr 1;23(2):141-73.https://academic.oup.com/jigpal/article-abstract/23/2/141/648641
Cohen T, Widdows D. Embedding of semantic predications. Journal of biomedical informatics. 2017 Apr 1;68:150-66. https://www.sciencedirect.com/science/article/pii/S1532046417300527
Cohen, T, and Widdows, D. 2018. Bringing Order to Neural Word Embeddings with Embeddings Augmented by Random Permutations (EARP). In Proceedings of the 22nd Conference on Computational Natural Language Learning, pages 465–475, Brussels, Belgium. Association for Computational Linguistics. https://aclanthology.org/K18-1045/
Mower J, Subramanian D, Cohen T. Learning predictive models of drug side-effect relationships from distributed representations of literature-derived semantic predications. Journal of the American Medical Informatics Association. 2018 Oct;25(10):1339-50. https://academic.oup.com/jamia/article/25/10/1339/5052182
Ding X, Mower J, Subramanian D, Cohen T. Augmenting aer2vec: Enriching distributed representations of adverse event report data with orthographic and lexical information. Journal of biomedical informatics. 2021 Jul 1;119:103833 (IMIA Yearbook best NLP papers of 2022) https://www.sciencedirect.com/science/article/pii/S1532046421001611.
Guo Y, Qiu W, Wang Y, Cohen T. Automated lay language summarization of biomedical scientific reviews. In Proceedings of the AAAI Conference on Artificial Intelligence 2021 May 18 (Vol. 35, No. 1, pp. 160-168). https://ojs.aaai.org/index.php/AAAI/article/view/16089
Cohen T, Pakhomov. S. A Tale of Two Perplexities: Sensitivity of Neural Language Models to Lexical Retrieval Deficits in Dementia of the Alzheimer’s Type. In Proceedings of the 58th Annual Meeting of the Association for Computational Linguistics, pages 1946–1957, Online. Association for Computational Linguistics. https://aclanthology.org/2020.acl-main.176/
Xu W, Wang W, Portanova J, Chander A, Campbell A, Pakhomov S, Ben-Zeev D, Cohen T. Fully automated detection of formal thought disorder with Time-series Augmented Representations for Detection of Incoherent Speech (TARDIS). Journal of Biomedical Informatics. 2022 Feb 1;126:103998. https://www.sciencedirect.com/science/article/pii/S1532046422000144
Burkhardt HA, Ding X, Kerbrat A, Comtois KA, Cohen T. From benchmark to bedside: transfer learning from social media to patient-provider text messages for suicide risk prediction. Journal of the American Medical Informatics Association. 2023 Apr 12:ocad062. https://doi.org/10.1093/jamia/ocad062