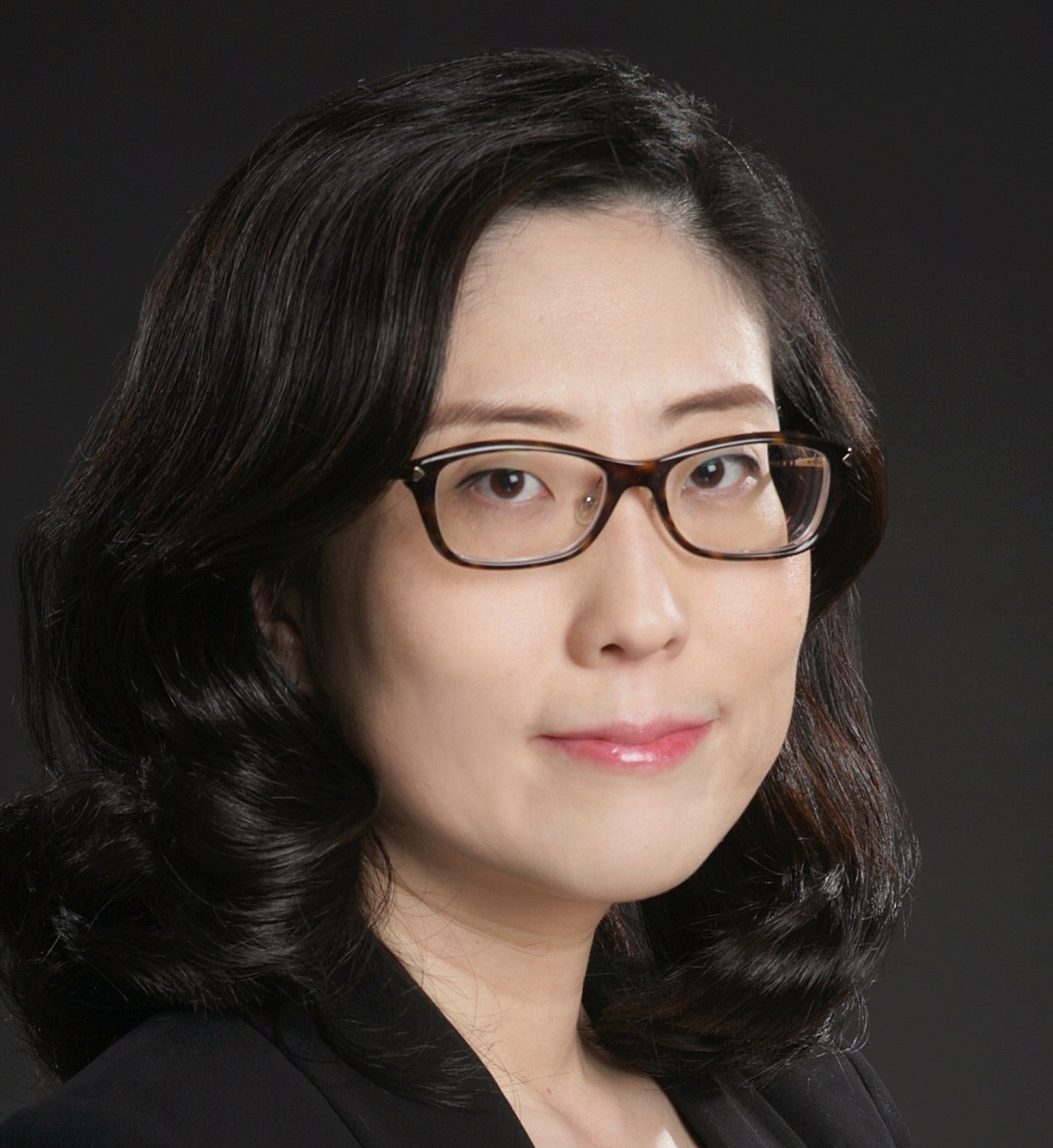
Interests:
Machine learning, computational biology, and clinical informatics.
Background:
Prof. Su-In Lee is an Associate Professor in the Paul G. Allen School of Computer Science & Engineering and in the Genome Sciences Department, and Adjunct Associate Professor in the Department of Electrical Engineering and in the Department of Biomedical Informatics and Medical Education at the University of Washington. She completed her PhD in 2009 at Stanford University with Prof. Daphne Koller in the Stanford Artificial Intelligence Laboratory. Before joining the UW in 2010, she was a visiting professor in the Computational Biology Department at Carnegie Mellon University.
Research:
Prof. Lee’s research seeks to develop interpretable machine learning techniques to learn from big data: (1) how the human genome or protein works, (2) how to improve healthcare, and (3) how to treat challenging and complex diseases such as cancer and Alzheimer’s disease.
Representative publications:
Su-In Lee, Safiye Celik, Benjamin A. Logsdon, Scott M. Lundberg, Timothy J. Martins, Vivian M. Oehler, Elihu H. Estey, Chris P. Miller, Sylvia Chien, Akanksha Saxena, C. Anthony Blau, and Pamela S. Becker. A machine learning approach to integrate big data for precision medicine in acute myeloid leukemia. Nature Communications 9, Article number: 42 2018.
Scott M. Lundberg, and Su-In Lee. A unified approach to interpreting model predictions. Neural Information Processing Systems (NIPS) 2017. Oral Presentation
Safiye Celik, Benjamin A. Logsdon, Stephanie Battle, Charles W. Drescher, Mara H. Rendi, David R. Hawkins, and Su-In Lee. Extracting a low-dimensional description of multiple gene expression datasets reveals a potential driver for tumor-associated stroma in ovarian cancer. Genome Medicine 2016 Jun 10;8(1):66.
Scott M. Lundberg, William B. Tu, Brian Raught, Linda Z. Penn, Michael M. Hoffman, and Su-In Lee*. ChromNet: Learning the human chromatin network from all ENCODE ChIP-seq data. Genome Biology 2016 Apr 30;17(1):82.
Maxim Grechkin, Benjamin A. Logsdon, Andrew J. Gentles, and Su-In Lee*. Identifying Network Perturbation in Cancer. PLOS Computational Biology 2016 12(5): e1004888.
Javad Hosseini, and Su-In Lee . Learning Sparse Gaussian Graphical Models with Overlapping Blocks. Neural Information Processing Systems (NIPS) 2016.
Naozumi Hiranuma, Scott Lundberg, and Su-In Lee. Cloud Control: Leveraging many public ChIP-seq control experiments to better remove background noise. The 7th ACM Conference on Bioinformatics, Computational Biology (ACM-BCB) 2016.
Benjamin A. Logsdon, Andrew J. Gentles, Chris P. Miller, C. Anthony Blau, Pamela S. Becker, and Su-In Lee (2015). Sparse expression bases in cancer reveal tumor drivers. Nucleic Acids Research 10.1093/nar/gku1290.